So far in this book we’ve looked at the role advertising technology platforms play in creating, running, and optimizing digital ad campaigns.
Now we’ll look at one of the most important elements that ties these two areas together: Data.
Data is the fuel that powers AdTech platforms and campaigns.
Specifically, data in digital advertising can be used for:
- Identification
- Targeting
- Reporting
- Attribution
- Campaign optimizations
Thanks to the rise of the Internet, advertisers and publishers now have access to enormous amounts of quality and generalized data sets that could never have been generated in the offline world. These data sets can give companies deeper insights into consumer behavior, identify trends, and improve campaign performance.
In this chapter, we’ll look at how companies collect data, the different types of data, the role of data platforms like data management platforms (DMP) and customer data platforms (CDPs), and what data is used for.
The Different Types Of Data: First-Party, Second-Party, And Third-Party Data
Not all data is the same, and each piece plays a different role for both advertisers and publishers.
First-Party Data
First-party data is considered the most valuable type of data for both advertisers and publishers because it is collected directly from people who have interacted with the brand, such as customers.
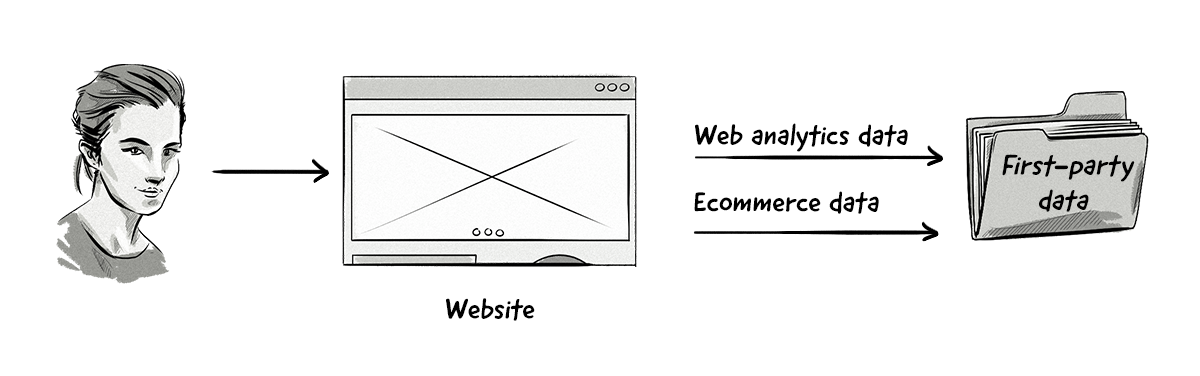
First-party data is often collected by:
- Ecommerce and offline transactions: Data about which products people have purchased and the value of orders, as well as personal information such as names, postal and billing addresses, email addresses, and phone numbers.
- Customer relationship management (CRM) systems: Data about people who have created an account with your business, downloaded a digital product (e.g. ebook), and purchased something from you. Just like with ecommerce data, this often includes names, addresses, phone numbers, and email addresses.
- Website and mobile app analytics: Data about which pages the user has browsed, videos they’ve watched, and other content interactions.
First-party data can come from online and offline sources (see the section below for more details).
Brands and advertisers use this type of data to convert visitors into customers and upsell products and services to existing customers.
Second-Party Data
Second-party data is sometimes referred to as partner data, as it is first-party information collected by one company and sold or traded to another.
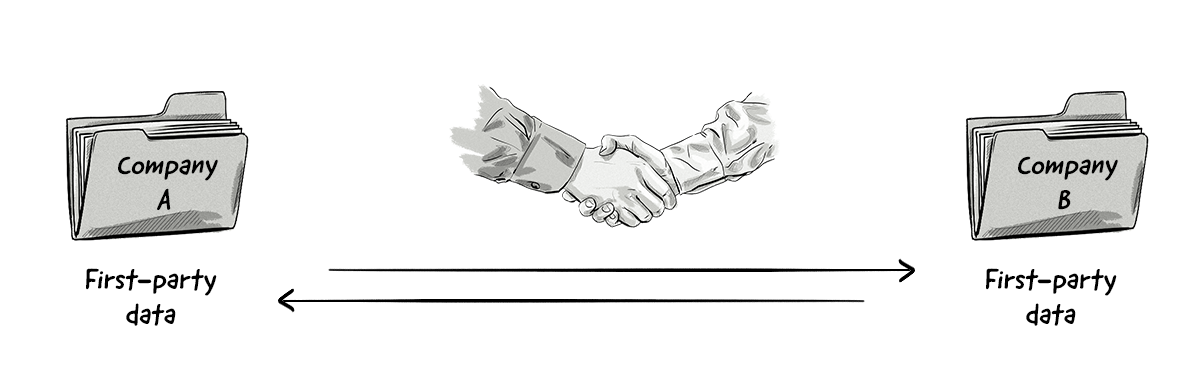
A typical partnership involves two non-competing companies with similar audiences.
For example, a hotel chain could partner with an airline and buy or trade the airline’s first-party data. The hotel chain could use the airline’s data to run targeted ad campaigns and display ads promoting their hotels to the airline’s customers.
This partnership would benefit the hotel chain, as accommodation is often something people search for when booking flights.
The partnership could be one-way (i.e. the hotel chain buys the airline’s first-party data), or the hotel chain and airline could set up a data-trade deal where they share information with each other.
This would allow the airline to display ads and messages to the hotel chain’s customers as well.
As the airline collects a lot of value first-party data, they could partner with many other types of companies, such as with exclusive brands that could target the airline’s high-income customers with luxury products like watches and jewelry.
While first-party data is more valuable, as it contains people who either are existing customers or have expressed interest in becoming one, second-party data allows brands and advertisers to reach a new, untapped group of potential customers.
Third-Party Data
In terms of value, third-party data comes in last place. It is neither collected from the advertiser or publisher directly and isn’t provided via a data partnership agreement.
However, third-party data still adds value to marketing and advertising campaigns and provides a couple of advantages over first- and second-party data, with the ability to reach a much bigger audience being the main one.
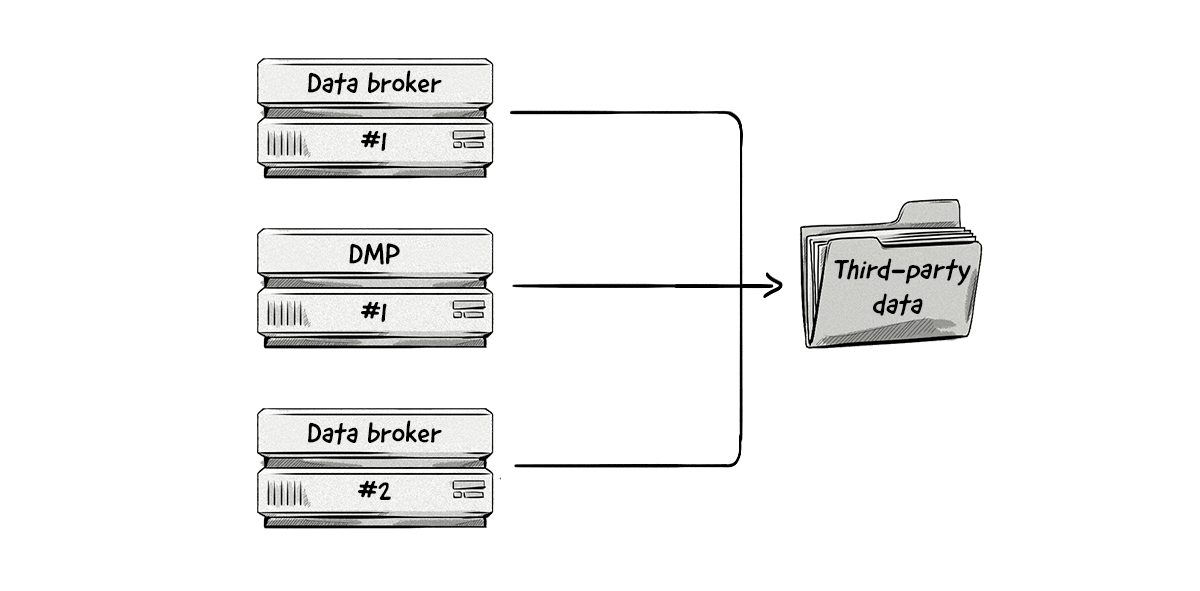
Third-party data is usually supplied by data brokers or is added as a layer by a DMP vendor.
Many publishers and merchants monetize their data by adding third-party trackers to their websites or tracking SDK to their apps and passing data about their audiences to data brokers and DMPs.
This data can include a user’s browsing history, content interactions, purchases, profile information entered by the user (e.g. gender or age), GPS geolocation, and much more.
Based on these data sets, data brokers can create inferred data points about interests, purchase preferences, income groups, demographics and more.
The data can be further enriched from offline data providers, such as credit card companies, credit scoring agencies and telcos.
From there, data brokers and DMP vendors can create audience segments.
Audience segments are made up of user profiles, which consist of various pieces of information, such as interests, location, and demographic information (e.g. gender and age).
Check out the Data Normalization and Enrichment in a DMP section located later in this chapter for more information about user profiles and audience segments.
A Comparison Of The Value Of First-, Second-, And Third-Party Data
Below is a comparison that illustrates how the different types of data stack up against each other.
First-party data | Second-party data | Third-party data | |
---|---|---|---|
Relevance and transparency How closely connected is the audience to the advertiser’s target audience and how transparent is the quality of the data? |
★★★
First-party data is made up of consumers that either are existing customers or who have engaged with a brand or publisher, meaning the audience is usually already part of the advertiser’s target audience. |
★★☆
Second-party data from one partner quite often contains audiences that share similar characteristics to the second partner’s target audience. |
★☆☆
Because third-party data is collected and aggregated from different sources, the direct connection between an advertiser and user is lost. This means the relevance is often low. |
Accessibility How easy is it for an advertiser to collect? |
★★★ As first-party data is collected directly from the brand or publisher’s website or app, it is the easiest type of data to collect. |
★☆☆ Second-party data requires data-sharing agreements and systems integration for each partner, thus making it a time-consuming activity. |
★★☆ Once an integration with a DMP or a data broker has been established, you can buy data sets on demand without the need for additional implementation. |
Competitiveness What competitive advantage could this type of data provide? |
★★★ As first-party data is exclusively available to the brand or publisher, it can be used for high-converting activities, such as content and ad personalization. |
★★☆ Second-party data can be shared exclusively, meaning it can be used as a competitive advantage over other companies offering the same products or services. |
★☆☆ As third-party data is usually widely accessible, many companies have access to the same pieces of data, meaning third-party data provides less of a competitive edge. |
Reach How many people could an advertiser reach by using this type of data? |
★☆☆ First-party data is limited to the visitors of the website (i.e. their online audience) and existing customers (e.g. offline CRM data). |
★★☆ Although it offers more reach than first-party data, second-part data is still limited to the partner’s audience. |
★★★
As data brokers and DMPs aggregate data from multiple partners, they have data on almost every user on the Internet. |
As you can see from the table above, first-party data is by far more valuable than second- and third-party data in most areas.
Where Is Data Obtained?
Brands, advertisers, marketers, and publishers gather data from a range of online and offline sources.
Online Sources
Companies collect huge amounts of online data from a variety of sources, mainly:
- Analytics tools
- Customer-relationship management (CRM) systems
- Enterprise resource planning (ERP) systems
- Marketing automation platforms
- Mobile and web apps
- Campaign analytics
Offline Sources
Offline data can be collected from the following sources:
- Point of sale (POS)
- Offline CRM and ERP systems
- Transactional data
As all of the above data is collected directly from the user, it is classified as first-party data.
The types of data listed above are usually stored in various databases, which can either be the advertiser’s or marketer’s databases, or the software vendor’s databases.
Combining Online and Offline Data Together
Companies that collect both online and offline data would combine them together to get a clearer picture of their customers and audience.
For large companies, such as retailers, integrating their offline and online records is not an easy task, but once it’s done, it can prove valuable as it provides several business advantages.
If, however, a company collects small amounts of offline data (e.g. only email addresses), it is possible to just import the data into a database or DMP. But if a company collects large amounts of offline data, then they will need to onboard it into a data platform like a DMP or CDP.
See the First-Party Data Onboarding section below for more information.
The Data Fragmentation Problem
While collecting vast amounts of data from multiple sources allows advertisers to improve campaign performance, the data is often stored across multiple tools and platforms. These individual databases are often referred to as data silos.
What is a data silo?
A data silo is a collection of data controlled by one department (e.g. sales) and isolated from other departments within an organization.
The main disadvantage of having data silos is that the data from different departments (or systems) cannot be integrated together, which restricts the data’s full potential from being realized.
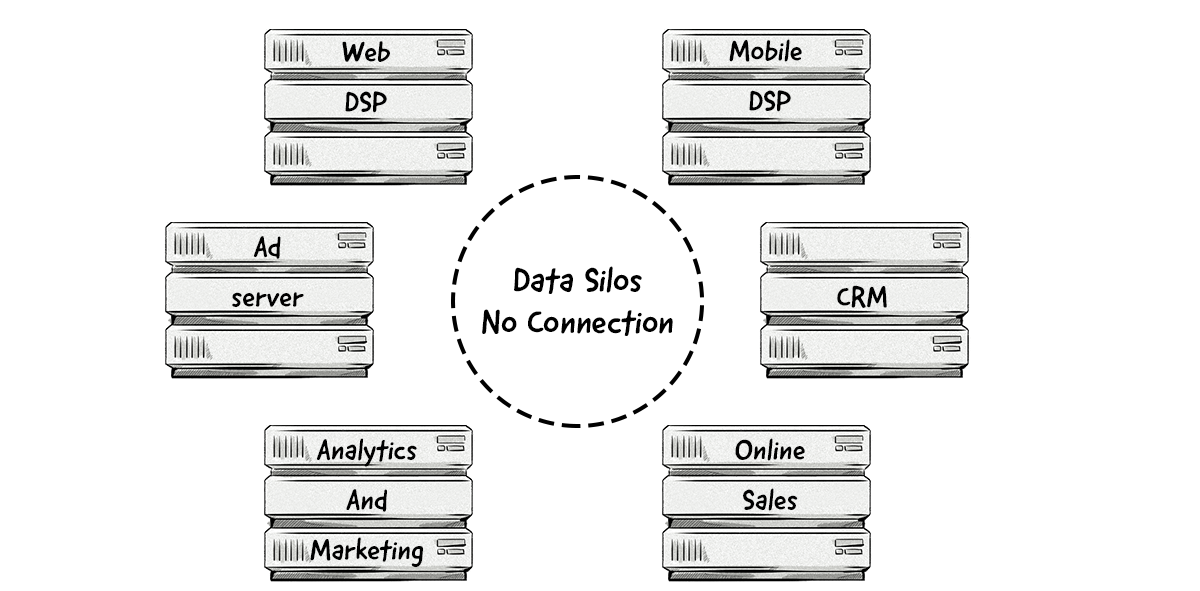
Having data stored in different silos means that advertisers can’t see the full picture of their target audience or campaigns’ performance, which often leads to poor decision-making, missed opportunities, and ad waste.
The solution to this problem is a data management platform (DMP).
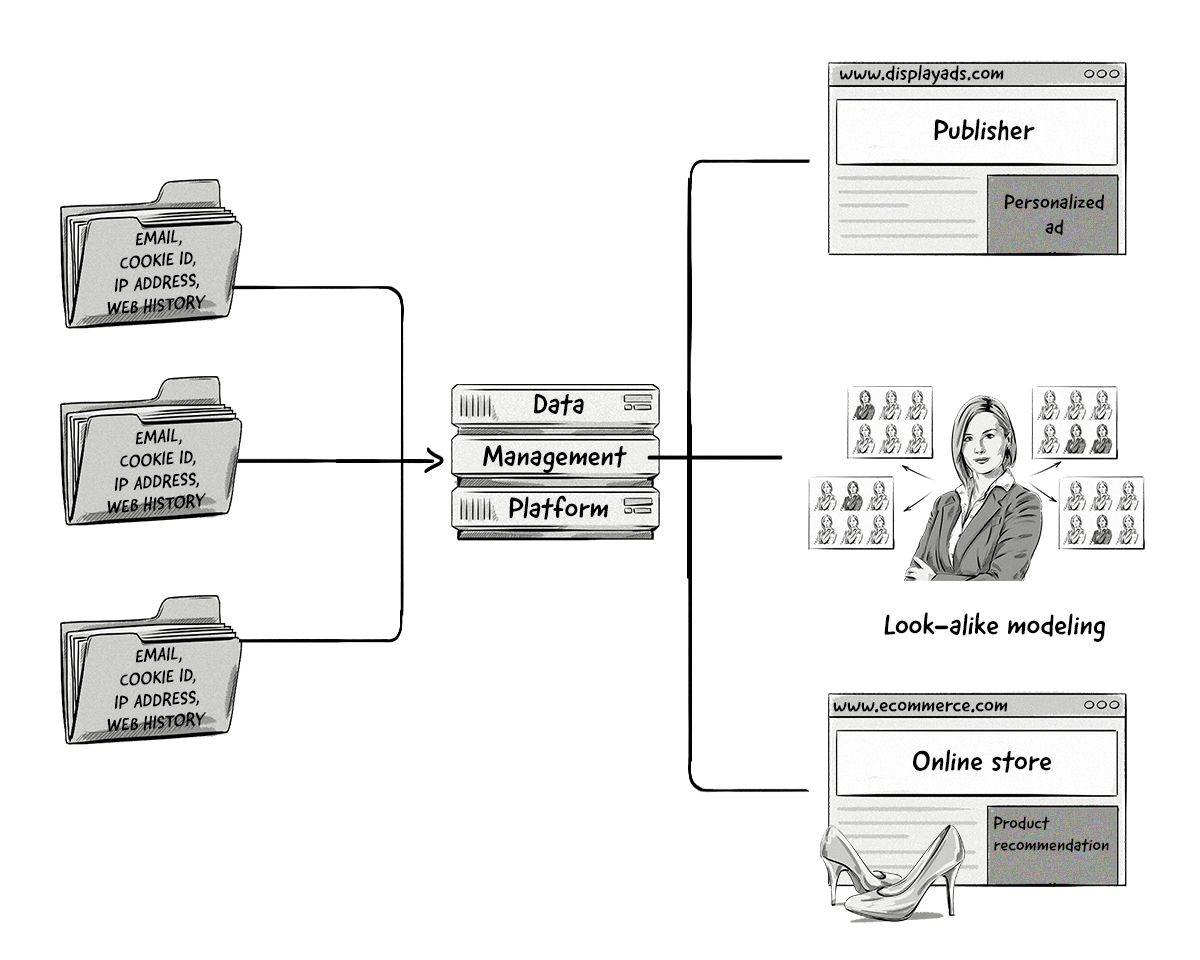
Data Management Platform (DMP)
As we’ve touched on previously, a data management platform (DMP) is a technological platform used to collect, store, analyze, segment, and activate data.
In this section, we’ll look at the main functions of a DMP and uncover some of its potential use cases.
Here’s a look at the processes and components of a DMP:
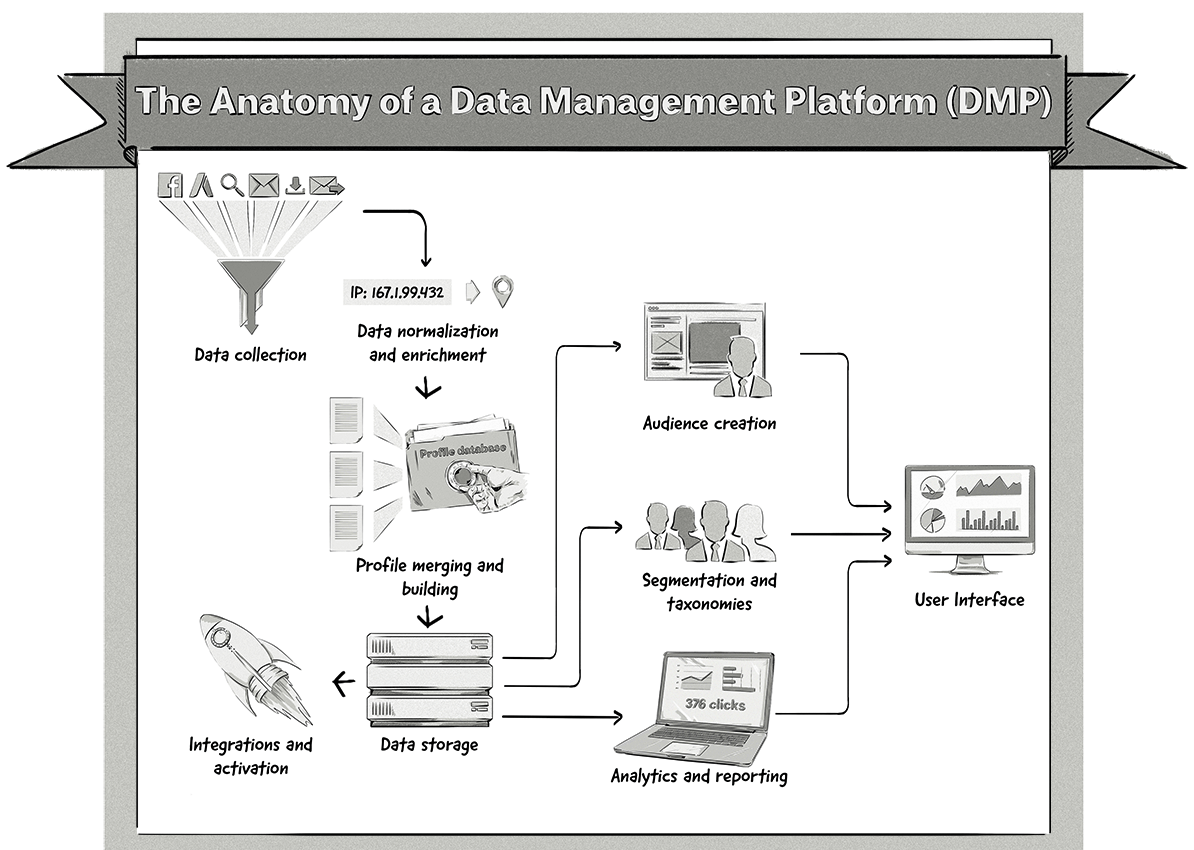
Let’s take a closer look at these components and processes.
It’s worth noting that the below processes can also be carried out by other data platforms like customer data platforms (CDPs), which we cover towards the end of this chapter.
Data Collection in a DMP
Collecting data can be done in a few different ways, depending on where the data is stored.
Pixels and Tags
Probably the simplest way for a DMP to collect first-party data is by adding a 1×1 transparent pixel (also known as a tag or tracking pixel) to your website.
The pixel itself is just a piece of HTML. When the pixel loads on a page, it sends off a request to the DMP to retrieve the 1×1 transparent image.
Once the DMP has returned the 1×1 pixel, it can assign a cookie to the user and store it in their browser. The information in the cookie can then be passed to the DMP.
Piggybacking
Piggybacking is when we insert a single master pixel on a site’s pages which can either contain or trigger multiple tracking pixels from various sources and networks that are not placed directly on the site.
When the master pixel loads, it subsequently loads the other pixels.
The images below illustrate the piggybacking process:
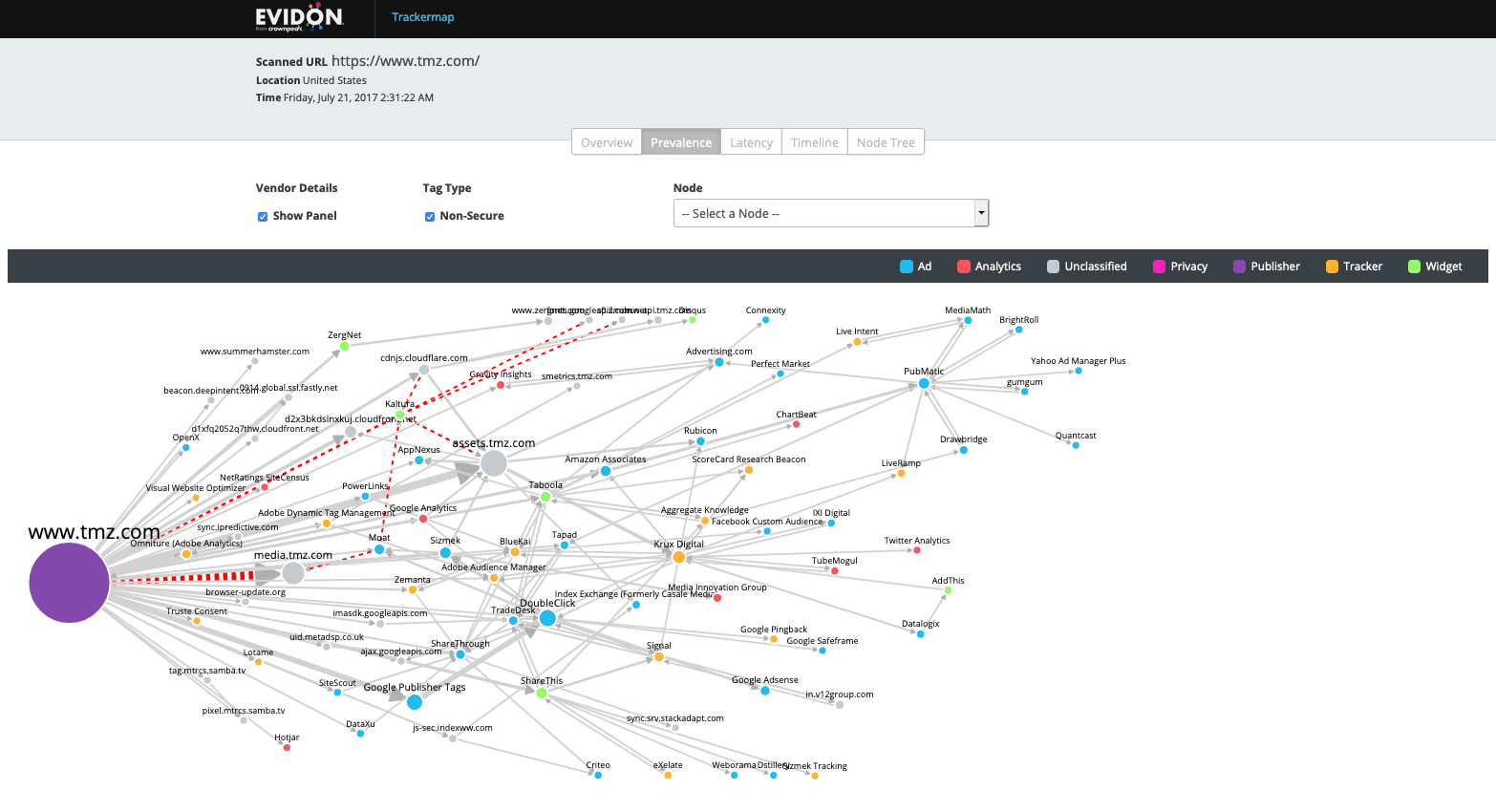
Piggybacking advantages | Piggybacking restrictions |
---|---|
One pixel can consolidate all third-party pixels. | Image pixels can only piggyback off one image pixel. |
A single pixel keeps everything clean and organized. | JavaScript pixels can actually piggyback off an unlimited number of JavaScript and image pixels, but too many piggybacked pixels could slow down the user’s browser. |
Data is tracked more accurately across all marketing channels. | Insecure (http://) pixels can only be placed on an insecure page — i.e. they can’t be placed on pages with https://. |
It reduces the need for web developers to be involved in implementing pixels on a site. | Publishers don’t have control over the piggybacked pixels, which can lead to problems around privacy and complying with data protection laws like the GDPR. |
Tags
Tags are pieces of JavaScript or an iframe. Just like with pixels, tags are added to a website and when loaded, send a request to a DMP. The DMP responds to the request and places a cookie in the user’s browser and collects data.
Sometimes, publishers will use a tag management system (TMS) to control and manage the various JavaScript snippets and pixels they have on their website. These tags and pixels are placed in a container which is inserted into a website’s pages, usually directly under the opening body element <body>.
The main benefit of a tag manager is that publishers can easily add, remove, and modify their HTML tags, JavaScript snippets, and pixels from a single user interface, rather than having to ask their web developers to make manual changes in the website’s HTML.
Application Program Interface (API)
APIs are used to exchange data between web servers and a DMP. This type of data exchange is ideal for companies that have a number of data silos as it allows them to efficiently collect data from different databases.
This form of data collection is also referred to as a server-to-server integration.
First-Party Data Onboarding
First-party data onboarding involves taking a company’s offline customer data and integrating it with their online customer data.
So, for example, a company could have the following customer data in their offline database:
- Names
- Residential addresses
- Phone numbers
- Email addresses
- Dates of birth
- And all other data they have about customers in their offline customer relationship management (CRM) and transactional systems.
They could then onboard it with the data they have in their online databases, such as:
- Data from their web-analytics tools and ad servers.
- User account information (e.g. account information from the company’s online payment system).
- Any other online information the company has collected about the customer, including the same information they’ve collected offline — e.g. name, email, and residential address.
Depending on the amount of offline data a company has, they might just be able to import the data as a CSV file. But if they have a large amount of data, which is often the case, then they would likely need to use a data onboarding platform like LiveRamp.
Here’s a list of some of the most common data-onboarding platforms on the market:
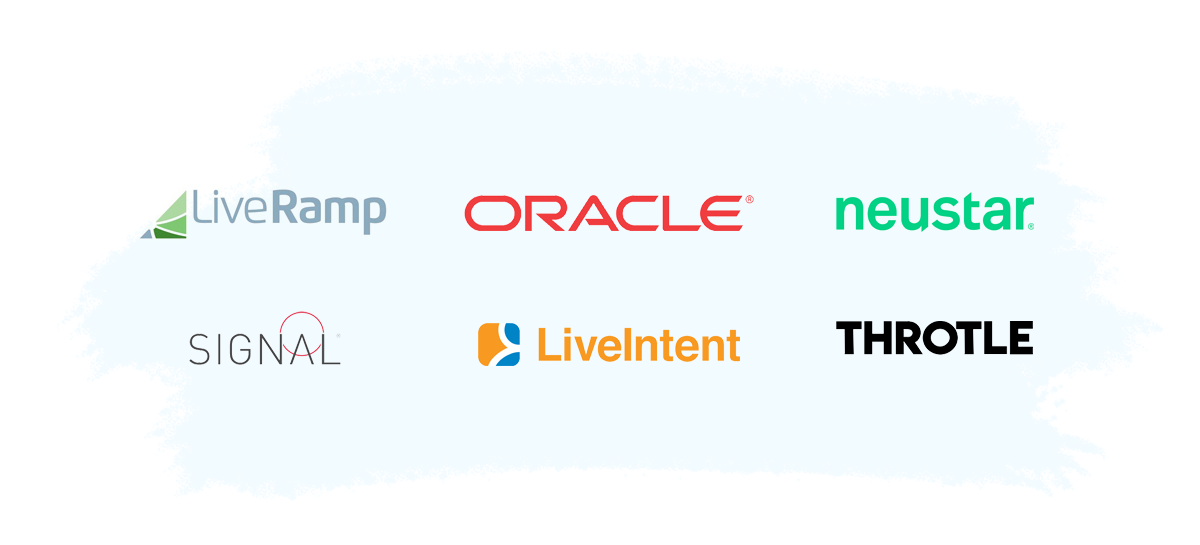
The general process involves a company uploading offline data with an onboarding platform, anonymizing it to remove any personally identifiable information (PII), e.g. email addresses, names, physical addresses, etc., and matching the offline data with the company’s online data.
How can data be anonymized?
Below are the most common ways to anonymize data and remove PII:
Hashing – irreversibly converting data into a non-human readable value.
Encryption – making the data accessible only to those with the decryption key.
Generalization – replacing a specific category with a more general one, for example, changing a user’s age from 42 to an age range of 40-49, Starbucks Coffee Shop at Wrocław Market Place to a coffee shop.
Suppression – replacing attributes, or parts of them. For example, changing a zip code from 44340 to 44***, location from 51.1088316,17.032966 to 51.1******,17.0******
Adding noise – adding random values to numeric attributes in a way that their average remains unchanged.
Swapping the data – exchanging certain fields of one record with the same fields of another similar record, for example, swapping the ZIP codes of two records.
The definition of “anonymized data” varies among companies and countries.
In the USA, it generally means removing personally identifiable information like names, postal addresses, and email addresses.
However, under certain privacy laws, like the EU’s GDPR, the term anonymized data means any data that can’t be used to identify a person. So, even if a company just collects cookie IDs, device IDs, and IP addresses, this data is still classed as personal data under the GDPR because AdTech and MarTech platforms can still identify users using those pieces of data (e.g. identify returning visitors).
Data anonymization is done to mitigate exposure of PII, such as in the event of a data breach, and to comply with certain data protection and privacy laws.
How Does First-Party Data Onboarding Work?
While each data onboarding platform will handle the process differently, the basic principle is the same:
- Companies upload their offline first-party data to the onboarding platform by importing CSV, CRM, and XLS data files
- Through an anonymization process, the onboarding platform transforms the data to remove any personally identifiable information (PII).
- The offline data is then matched with the online data with the help of identifiers. For example, if a company has collected a customer’s email both offline and online, the onboarding platform would match the two sources of data via the common email address.
Here’s a visual representation of the data onboarding process:
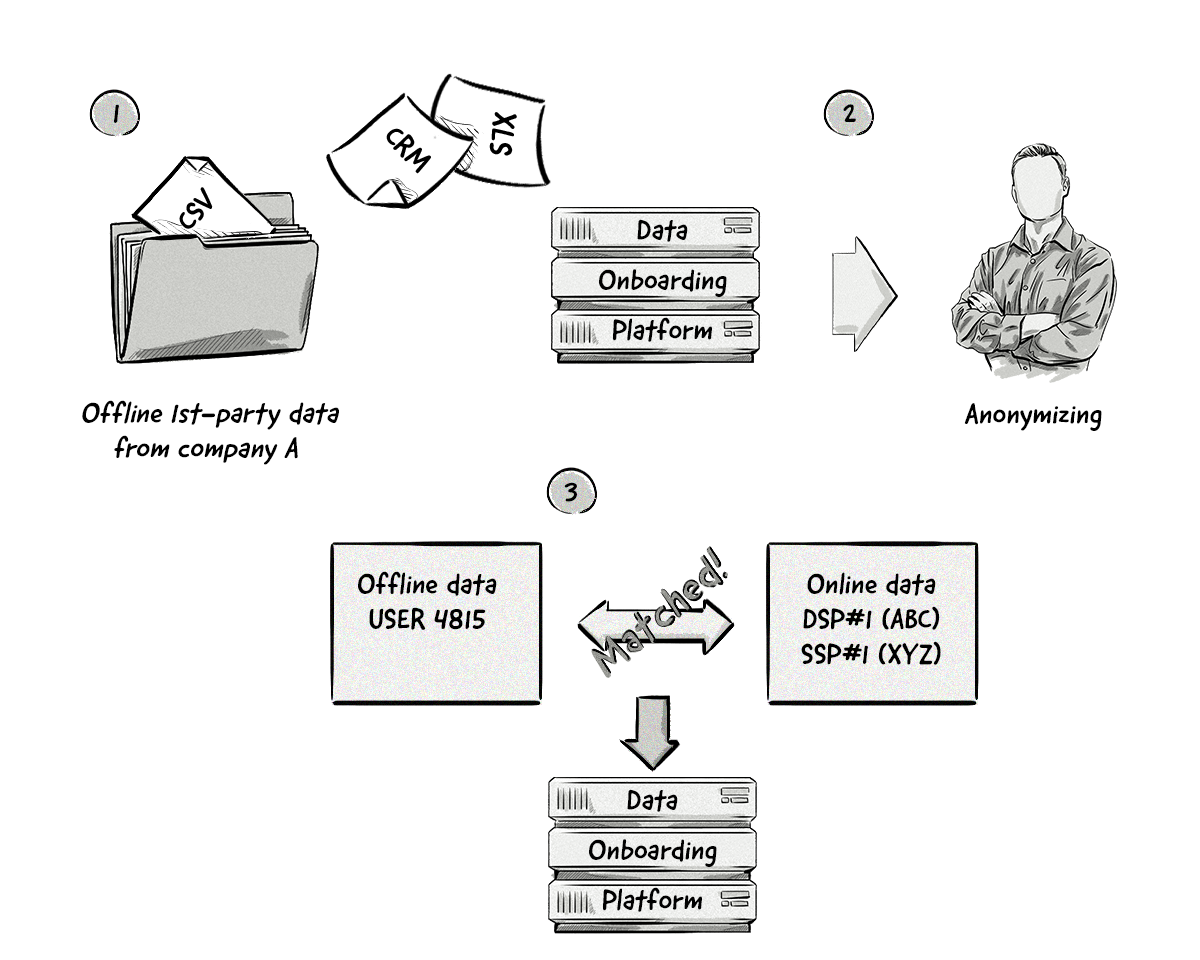
Data Onboarding With Google AdWords and Facebook Ads
Due to the vast amounts of data that is being onboarded via companies like LiveRamp, the process can be quite complex and take a number of days to complete.
Other companies, like Google and Facebook allow advertisers to upload simpler offline and online data sets (typically just an email address) to their platforms and use it for targeting and retargeting. Although this method doesn’t offer the same scale as data onboarding via the companies above, it’s often a suitable option for small- and medium-sized companies.
Google Ads
Google’s Customer Match service allows companies to upload their audience data to Google Ads and target those customers, as well as related audiences, across Google’s properties, including the Search Network and Google Shopping, YouTube, Gmail, and the Display Network.
The data that can be uploaded to Customer Match includes:
- First name
- Last name
- Country
- Zip code
- Phone number
Facebook Ads
Facebook’s Custom Audiences works in a similar way to Google’s Customer Match.
This service allows companies to not only upload customer email lists to Facebook Ads for targeting on the Facebook platform, but to also place a tracking pixel on their website and/or app. From there, the pixel will track that user and then display a customized ad or offer to that user on Facebook.
Data that can be used to create a custom audience in Facebook includes:
- Phone number
- First name
- Last name
- City
- State or province
- Country
- Date of birth
Data Normalization and Enrichment in a DMP
Once the data has been collected, it’s time to normalize it.
The data-normalization process can include a number of the following actions:
- Gathering IDs from web cookies.
- Deleting redundant or useless data.
- Transforming the source’s data schema to the DMP’s data schema.
- Enriching the data with additional data points, such as geolocation and OS/browser attributes.
The data normalization and enrichment stage provide two main benefits:
- It organizes the various data sets into a common format.
- It improves data value and quality.
During the normalization and enrichment stage, each user will be assigned a unique ID and given different attributes, which will play a key role in the segmentation stage.
These attributes can include:
- Age
- Gender
- Location
- Browser history
- Interests
- Purchase history
Profile Building and Merging
A profile is a set of data collected from events tracked by a DMP. It represents a user and may contain the following pieces of information:
- profile id
- cookie id (list)
- hashed email (list)
- sid / uuid (list)
- country (last seen)
- name (nullable)
- device_type (last seen)
- device_vendor (last seen)
- device_os (last seen)
- browser_vendor (last seen)
- gender (nullable)
- company (nullable)
- company size (nullable)
- matching ids (list)
In some cases, a profile will be created containing only a few pieces of data (e.g. cookie id, device_type, and device_os) and will be extended when more data becomes available — i.e. profile building.
When a DMP receives new events containing a known piece of data (i.e. one that is in the DMP), then it is added to the relevant profile.
On the other hand, if an input event contains a new piece of data (i.e. one that isn’t in the DMP), then a new profile is created.
It’s quite often the case that two profiles contain the same pieces of data (e.g. cookie id).
If this occurs, the DMP will have to perform an operation known as profile merging.
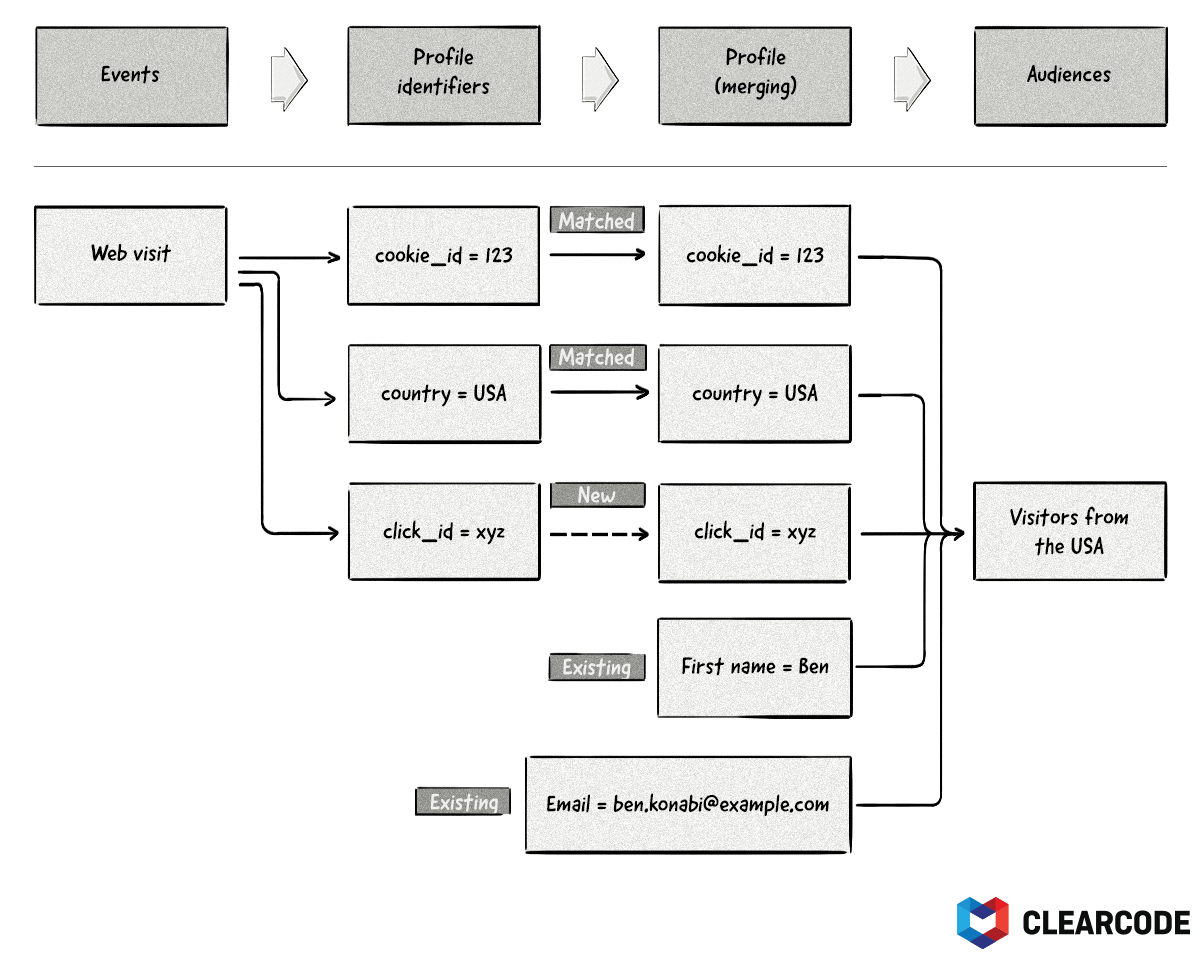
The goal of profile merging is to ensure that no profiles contain duplicate pieces of data and that no two profiles contain the same unique identifiers (e.g. cookie IDs and email addresses).
Let’s look at the following example:
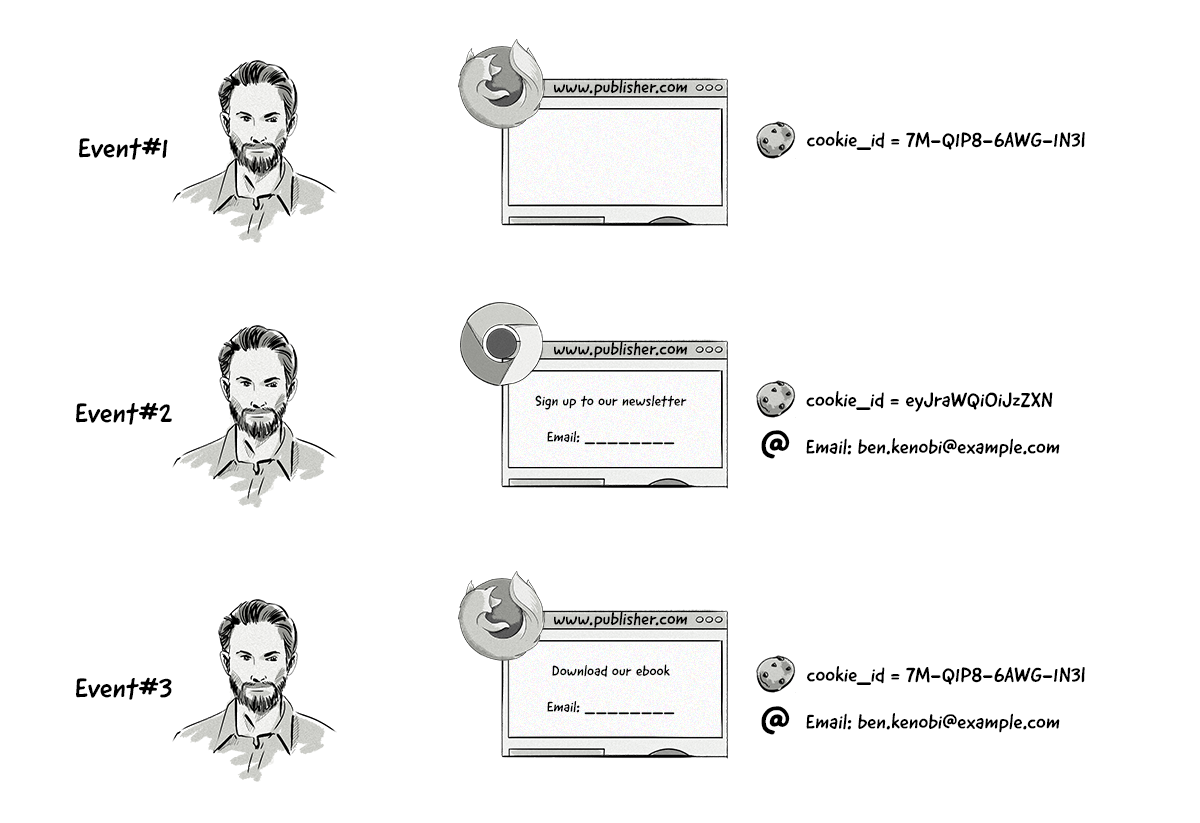
All three events come from the same user, but this isn’t known until the third event arrives in the DMP, meaning all three events would be treated as separate profiles.
For this example, all three profiles would be merged together into one profile.
Most DMPs would use a master ID — a single ID associated with one profile — to ensure accurate profile merging. Most often this would be a persistent ID, such as an email address.
When new events containing the master ID enter the DMP, all other data associated with the event will be added to that profile.
How to Merge Profiles Together
There are a few ways a DMP can merge profiles together.
Below we’ll list some of the main ways.
Overwrite existing IDs and attributes: This is one of the simplest ways to merge profiles as it simply replaces existing IDs with new ones as they enter the DMP.
Alphabetical sorting: This method sorts the values alphabetically and then uses the first value. For example, if there were 2 profiles with the names “Robert” and “Bob”, then “Bob” would be used as the name value because the letter “B” comes before “R”.
Timestamp sorting: With this method, the value that has the first or last recorded timestamp would be used.
In most cases, timestamp sorting will be the most desired method to use.
Wait-and-see sorting: A more complex approach would be to keep all values for reference until a different sorting method (e.g. timestamp) becomes available. Then you’d be able to see whether the assumption was correct and decide on the final values after the merging operation has finished.
Data Storage
Although the concept of storing data in a DMP seems rather simple, the actual technical implementation can be challenging due to the large amount of data being stored, as well as the need to move it to other areas and prevent data loss.
Data Taxonomies
Taxonomy in a DMP refers to the naming convention used for various pieces of data.
For example, instead of having two taxonomies like “user” and “visitor”, you could create or define one taxonomy (e.g. “user”) that represents both terms.
Below is an example of how an ecommerce store could structure its taxonomies:
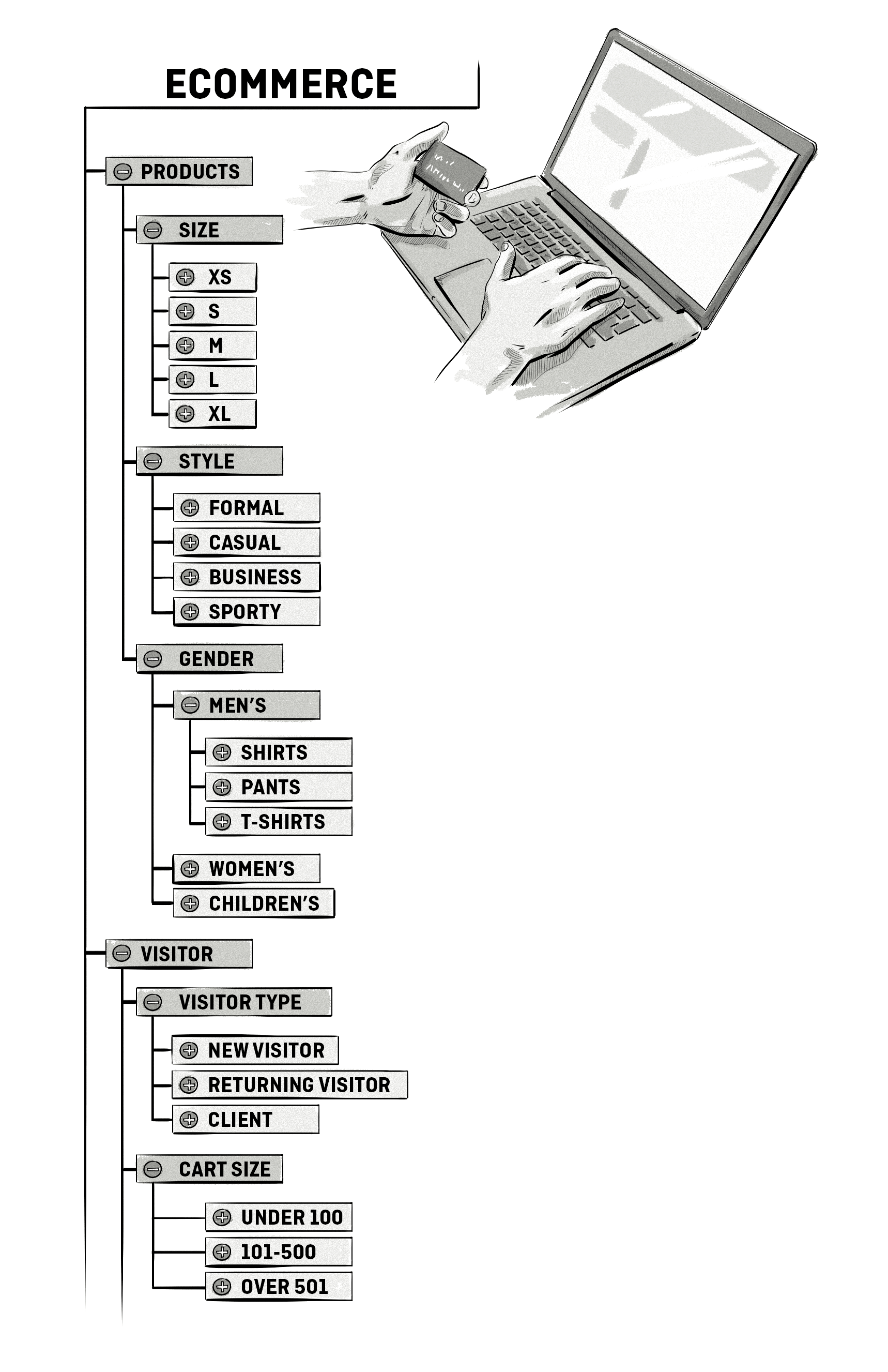
Audience Segmentation and Creation
Audience segmentation involves placing users into groups based on common characteristics, such as age, location, behavior, interests, and many others.
These segments form the basis for data activation, whereby these segments are used for a number of different purposes, such as for ad targeting and analytics.
See the Use Cases Of Data Activation With A Data Management Platform (DMP) section below for more details.
How A DMP Creates Audience Segments
To create audience segments, a DMP uses a series of conditions to filter the data and produce specific groups of users.
The conditions may include general information such as:
- Country, region, or city
- Device type
- Operating system
- Referral URL
And may also include more specific data about users’ behavior like:
- Events (button clicks, page-views, etc.)
- Conversions (downloads, purchases, etc.)
- Ads viewed
It could also contain demographic data, such as:
- Relationship status: In a relationship
- Interests: Gardening
- Age group: 35-39
- Gender: Male
- Home Value: Between $200k – $400k
- Annual income: Between $60k – $90k
Advertisers can then combine multiple segments to directly target the audiences they want to reach with their online advertising campaigns.
Here’s an example of what that might look like:
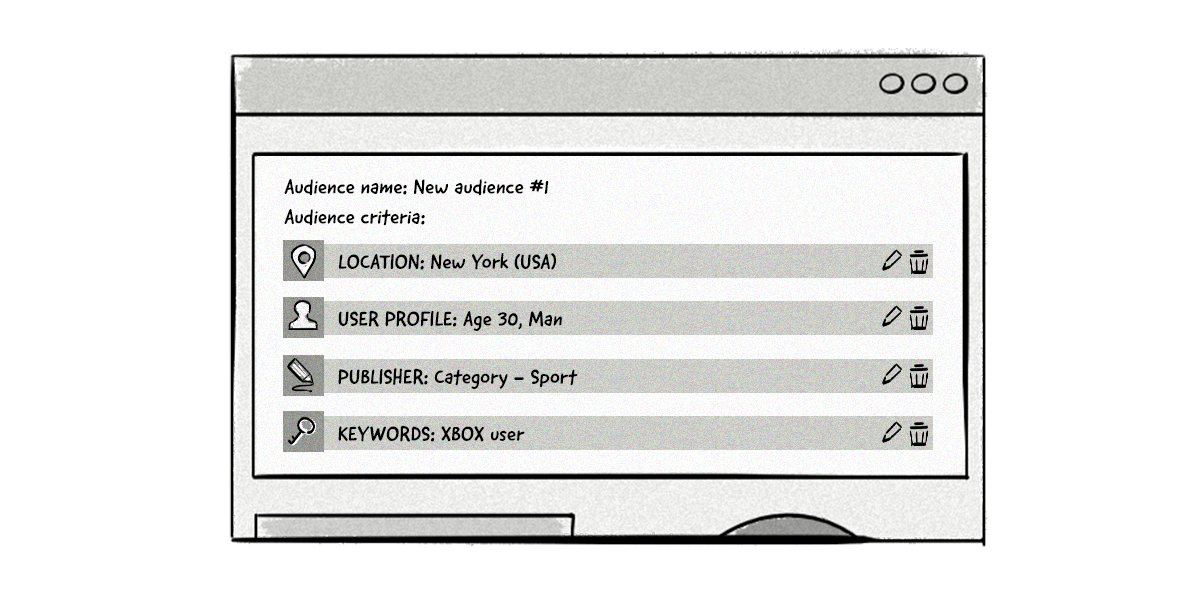
Apart from selecting which users to include in an audience segment, you can also add filters to exclude users from the segment and set the recency and frequency of certain actions.
For example, you could add users who have viewed your website at least 5 times (frequency) in the past 30 days (recency).
These two additional factors will go a long way towards defining your audience segments and their usefulness and can significantly impact the relevance and scope of the segments.
Relevance: Increasing the time frame for data points to be included. For instance, setting a time frame of “greater than 30 days” can add users who may be less likely to convert. However, raising the frequency to “at least 3 times” for certain event information can mean adding a user who is highly engaged and likely to convert.
Scope: Similarly, extending the time frame and reducing the frequency will broaden the scope of the audience, which would be useful for brand awareness but not for increasing conversions.
Once you’ve created audience segments, you can now activate your data.
Use Cases Of Data Activation With A Data Management Platform (DMP)
Data activation in a DMP is using audience segments for a range of different activities. It’s often considered the most important function of a DMP.
Below are the most common ways advertisers and publishers can activate their data and audience segments in their DMP.
Data Activation for Advertisers
Media Buying And Optimization
For advertisers and marketers, activating their data for digital media buying is one of the main use cases.
The main way to activate data for media buying is via cookie syncing.
We covered cookie syncing in a previous chapter: User Identification
First, the advertiser would start by integrating their DSP with a DMP. From there, the advertiser can create audiences and sync their cookies with the DMP’s cookies so they can identify their audience across multiple publishers.
When an advertiser (via a DSP) identifies a user who belongs to one of their audiences, they can show them an ad that is more personalized and relevant. Because this form of targeting is more dynamic than traditional targeting (e.g. showing iphone users the same ad), it often produces more clicks and conversions.
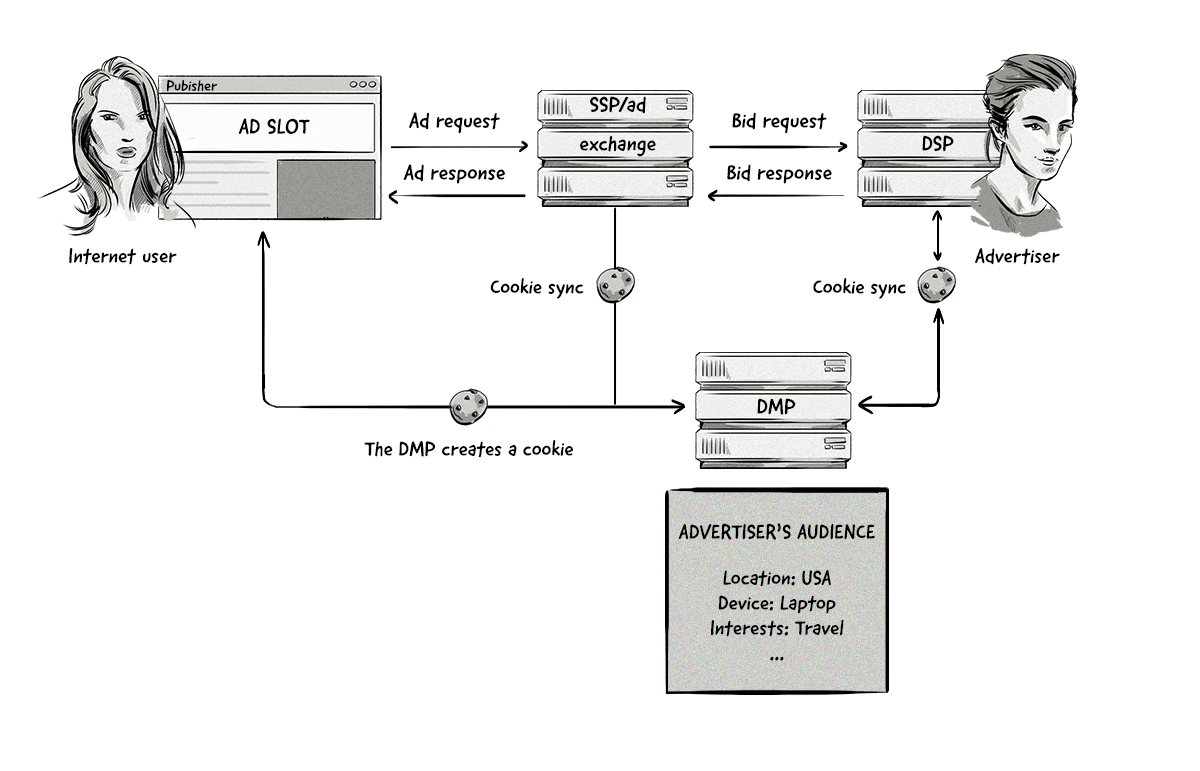
Brands and advertisers can also use a DMP to improve retargeting and dynamic creative optimization.
Another key part of activating data in a DMP for media buying is improving campaign performance and reducing ad waste.
DMPs can provide advertisers with detailed reports, allowing them to see the best and worst performing audiences, and make real-time optimizations to increase reach, performance, and optimize media spend.
Look-alike Modeling
Advertisers can use a DMP to perform lookalike modeling — a process that finds people that have similar characteristics to an advertiser’s target audience.
For example, an ecommerce store selling motorsport gear wanting to increase its audience could perform lookalike modeling to find new people who were interested in motorsports but hadn’t visited their store. They could define a set of criteria that matched their existing audience, such as location and interests, and then use a DMP to create those new audiences.
The ecommerce store could also place a pixel from a DMP on their purchase confirmation page to collect data about customer behavior across different websites. The DMP could analyze this data and look for similarities in behavior among the store’s existing customers. These similarities can then be used as the basis for creating new audiences.
How Does Look-alike Modeling Work?
Lookalike modeling analyzes data and uses algorithms to identify common characteristics and similarities in behavior.
Because the main goal of lookalike modeling is to find new audiences, it works best when it’s able to analyze data outside of an advertiser’s own database. For this reason, most lookalike modeling is done by DMPs that have collected large amounts of data from multiple sources.
To create a look-alike model, advertisers need to define the attributes and behaviors of their most valuable customers.
The stricter the look-alike model is (i.e. the more attributes), the better chance advertisers have of finding more people who match their target audience. This will improve the advertiser’s chances of receiving more conversions.
However, advertisers could be less strict with the look-alike model by defining fewer attributes and behaviors if their goal is to focus on reach and awareness rather than higher conversion rates.
Below is an example of a look-alike model that has tightly defined (more) attributes and behaviors, and one that has loosely defined (less) attributes and behaviors.
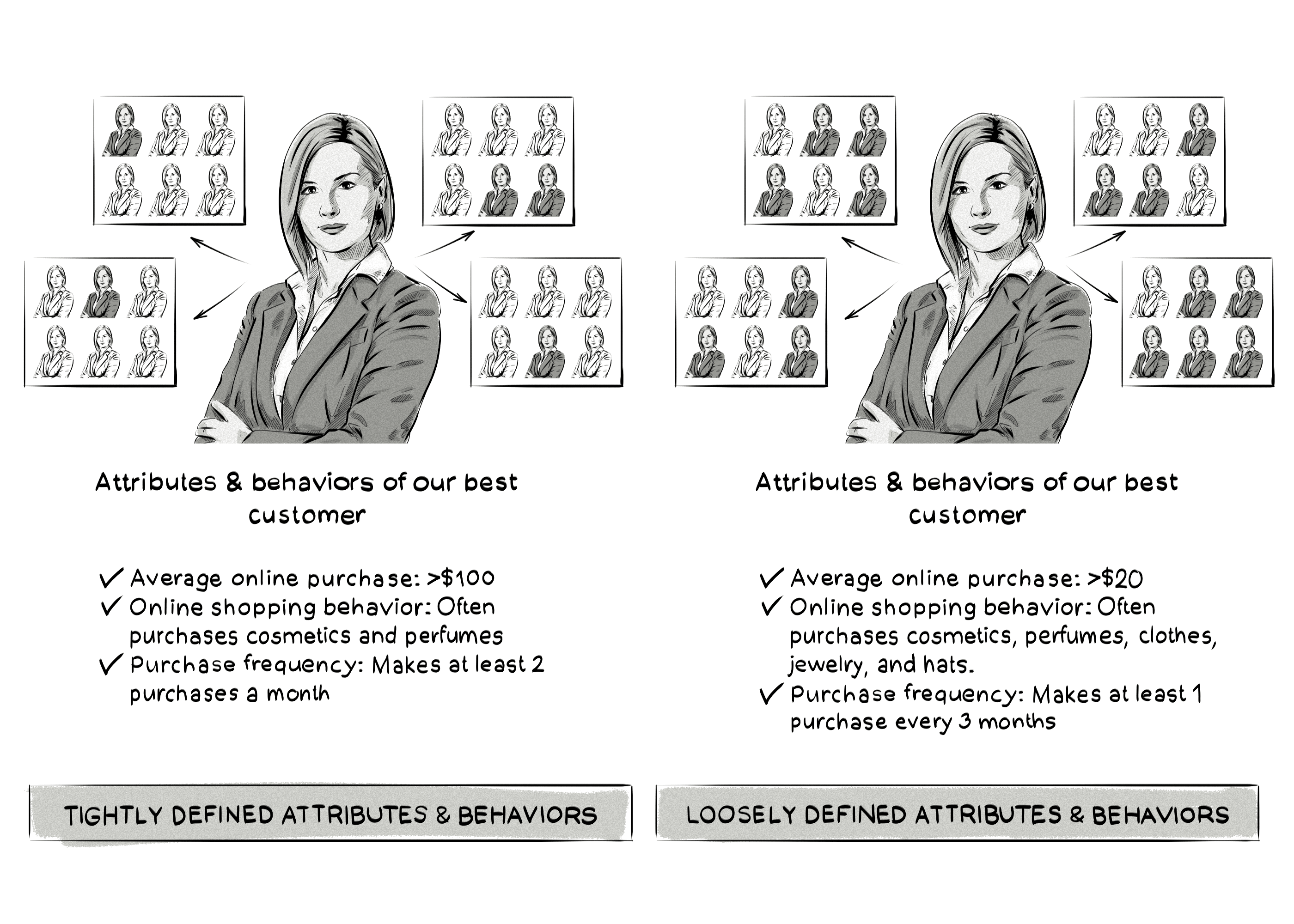
What Can Look-alike Modeling Be Used For?
The main use case of look-alike modeling is prospecting, which involves finding new potential customers and/or visitors.
However, it can also extend the reach of online advertising campaigns.
Let’s say you target audiences based on a set of attributes, such as age, gender and location.
By applying look-alike modeling to your campaigns, you can find similar customers who perhaps aren’t included in your current audiences because you don’t have enough data (e.g. we lack the attributes needed to make a match) or they don’t fit your current audiences (i.e. they consist of other attributes) but are still similar to your best customers.
Audience Intelligence
Advertisers can enrich visitor and customer data by matching and comparing their current audiences against third-party data sets and adding information about both customers and prospects, including their demographics, interests, income or purchase preferences, to their database.
This allows them to learn more about how people behave, fine tune their target audience, and identify what makes them convert.
Brands and advertisers can use audience intelligence to optimize and personalize certain areas of their site and campaigns to improve engagement and increase conversions.
For example, a travel website that promotes thousands of different hotels and resorts may identify a group of visitors who are less price sensitive, but require more flexibility and display more expensive offers with a free cancellation option to them.
Data Activation For Publishers
So far, we’ve explained the ways in which advertisers can use a DMP for data activation, so now it’s time to have a look at how publishers can utilize a DMP.
Similar to advertisers, the first step in monetizing their revenue is to create audience segments. From there, they can be used for a number of different use cases.
Here are the main ways publishers can activate their data.
Increase the Value of Their Inventory
Due to the large volume of user data that many medium- and large-size publishers collect, they can increase the value of their inventory by creating audiences and then offering them to advertisers.
The reason this benefits publishers is because advertisers will pay a higher price (e.g. CPM or CPC rate) if they know that their ad will be seen by the right target group and highly engaged users.
How are publishers able to pass these audience segments to advertisers?
There are a few ways publishers can make their audience segments available to advertisers and increase their ad revenue:
- Cookie syncing: A publisher’s SSP could sync cookies with a DMP or DSP to allow advertisers to bid on impressions that will be shown to a user in their target audience.
- Deal IDs in PMP deals: Private marketplace deals allow publishers to offer their most prized inventory to a select group of advertisers. By broadcasting their audience segments in PMP deals, publishers can earn even more money on their premium inventory.
- Segment ID in RTB auctions: A publisher’s SSP or DMP can pass segment IDs to DSPs during real-time bidding (RTB) auctions to help advertisers find their target audience.
Improve Engagement and Conversions With Content Personalization
Content personalization involves displaying content and recommendations that match users’ preferences based on their demographic information, interests, and the content they’ve consumed in the past.
The example below illustrates how using a DMP for content personalization works:
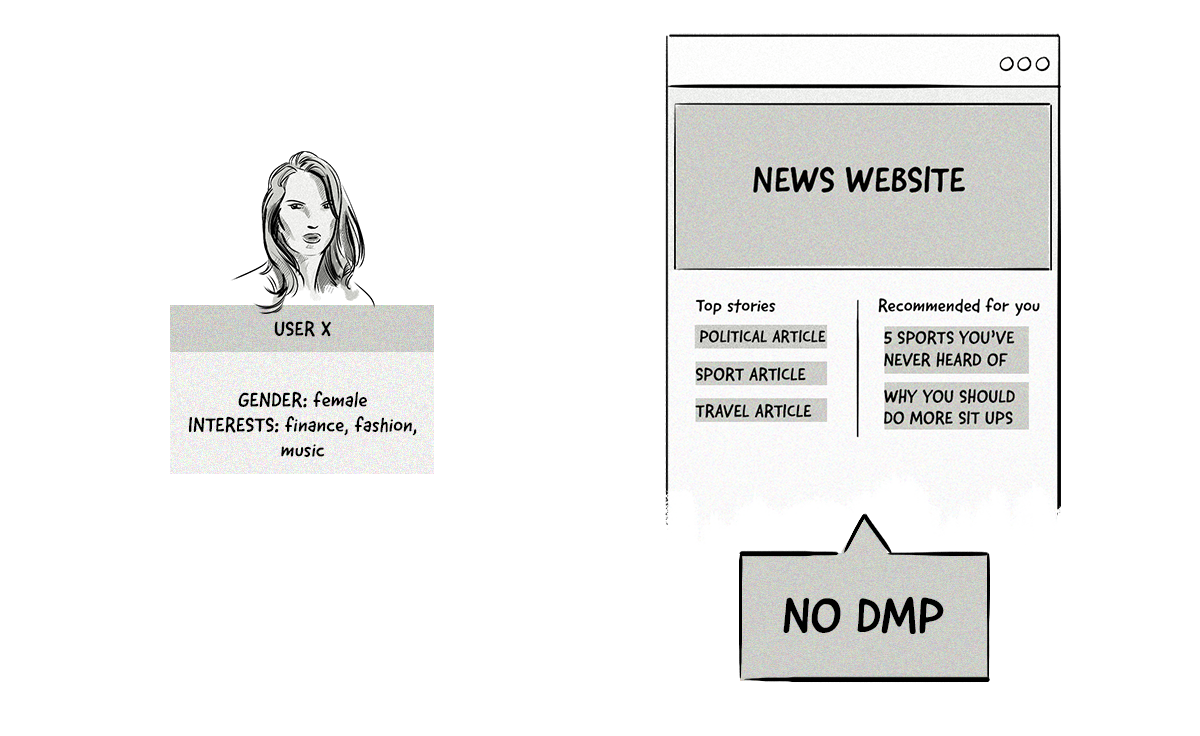
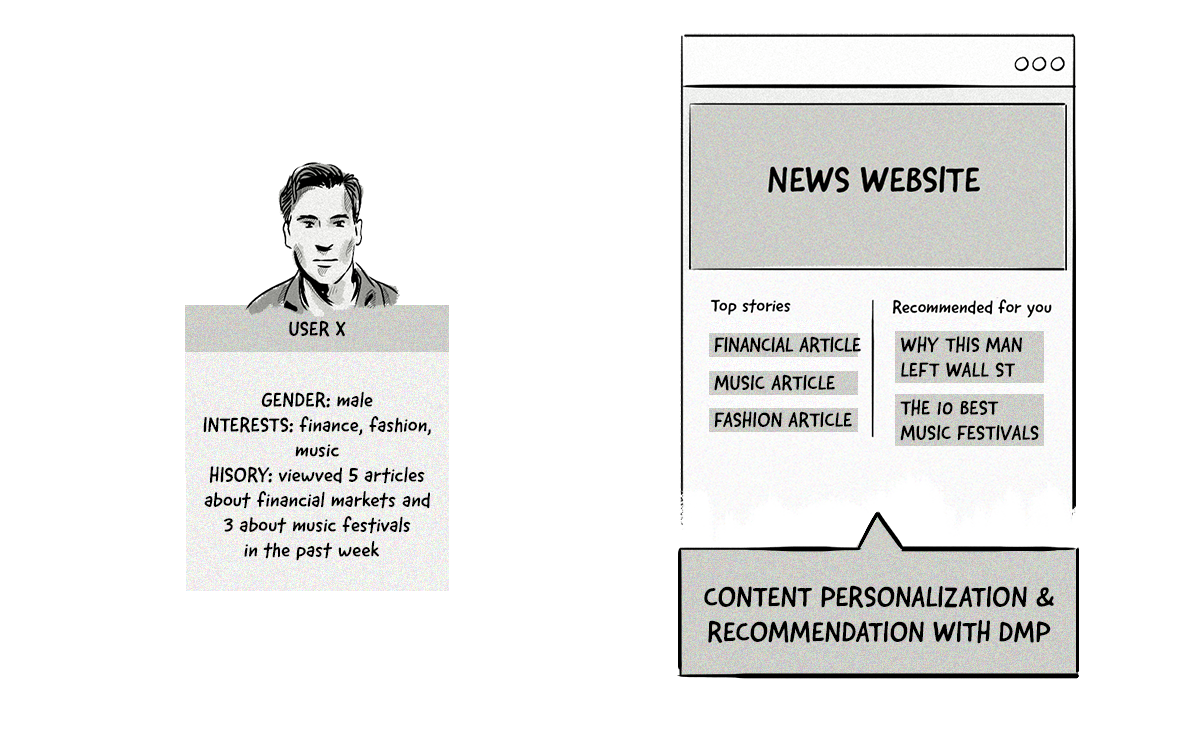
Audience Extension For Publishers
Publishers can use a DMP to create audience segments, which can be used for audience extension.
Audience extension involves a publisher creating audiences from their first-party data, which includes contextual and behavioral data like age, location, interests, web history, purchase history, click-based interactions, etc.
Publishers can then push these audiences to AdTech platforms, allowing advertisers to target these audiences across the Internet — not just target them on the publisher’s websites.
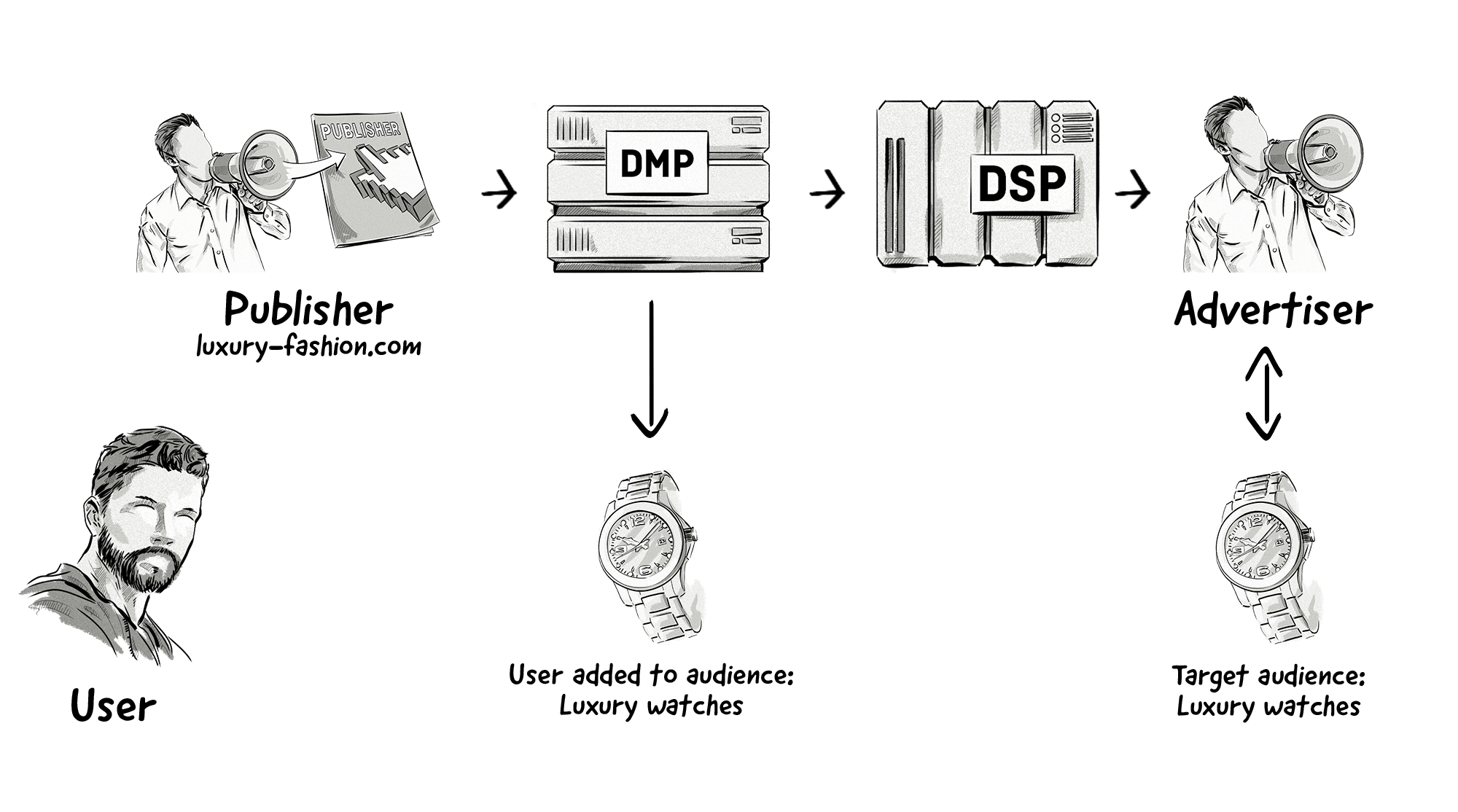
Here’s an overview of how audience extension works:
- The publisher’s DMP collects its first-party data and creates audience segments.
- The DMP passes on the publisher’s audience segments to advertisers via DSPs. This is done via cookie syncing.
- If an advertiser’s DSP identifies a publisher’s audience during an RTB auction originating from a different website, then it bids on that impression.
- If the DSP wins, the ad is displayed to the publisher’s audience but on a different site.
Audience extension is a win-win for publishers and advertisers — it allows publishers to create a new revenue stream and advertisers to find their target audiences across more websites.
Data Partnership
The publisher can set up a direct and exclusive partnership with another website, for example, a site that wants to run ad campaigns targeted at people living in the New York area that listen to the music online. The data used by the second website then becomes second-party data.
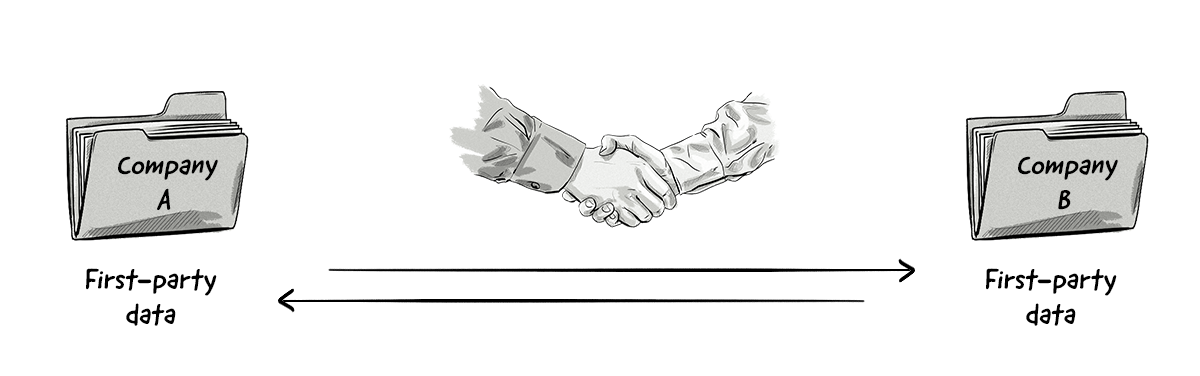
Selling Their Data
The publisher could also just sell the segments or anonymized user profiles to data companies (e.g. data brokers and DMPs).
The data broker could either use the segments created by the publisher or group the anonymized user profiles into demographic segments based on different characteristics, such as location, age, interests, etc.
The data broker would then resell the data to advertisers who want to direct their campaigns to a specific demographic group. The data in these user segments would now be classified as third-party data.
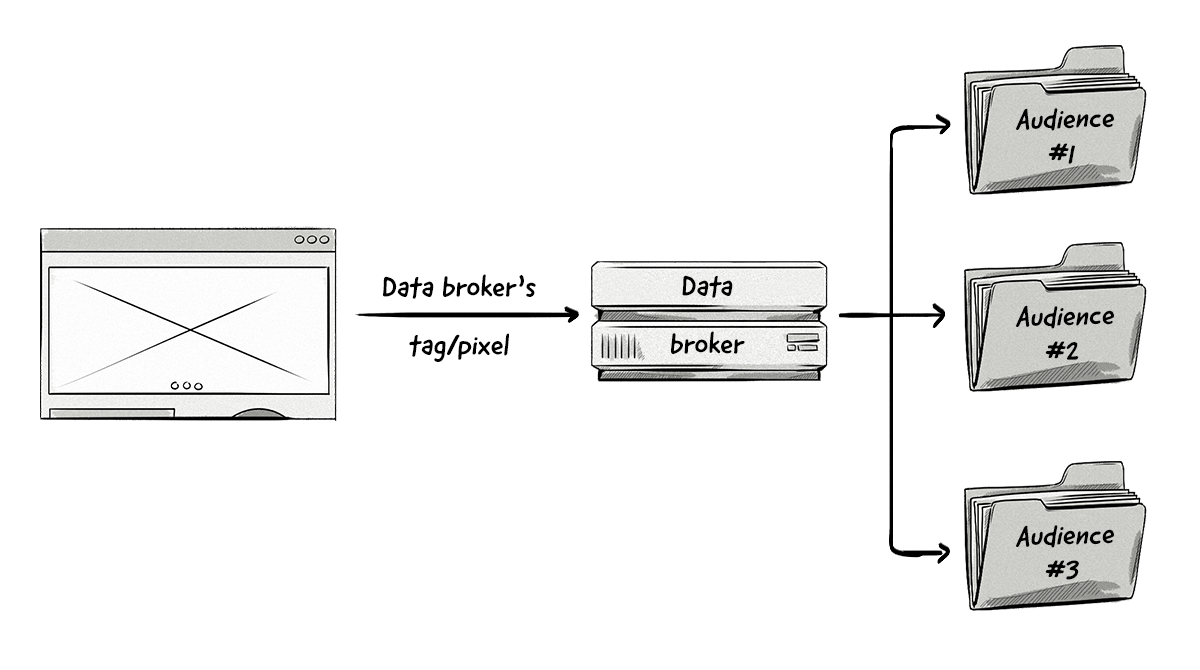
Compared to the partnership option, this monetization process is much less transparent. The publisher also loses its independence, as it is the data broker who decides which segments of data are shared and what kinds of segments are created from the publisher’s visitors.
Data Brokers and Integrations With Programmatic Media-Buying Platforms
While all companies that operate within the online advertising industry collect data, there are some companies that make a business only out of collecting and selling online consumer data. These companies are known as data brokers, or information brokers.
What Is A Data Broker?
A data broker is a company that aggregates user profiles from publishers and brands, combines them together, segments them, and then sells the segments to other companies to use in their online advertising campaigns.
There are several types of data brokers that operate in certain industries:
- Marketing and advertising: Improve ad targeting and campaign measurement.
- Identity verification and fraud detection: Help organizations like banks verify the identity of individuals.
- People search: Collect publicly available information about people from social media websites.
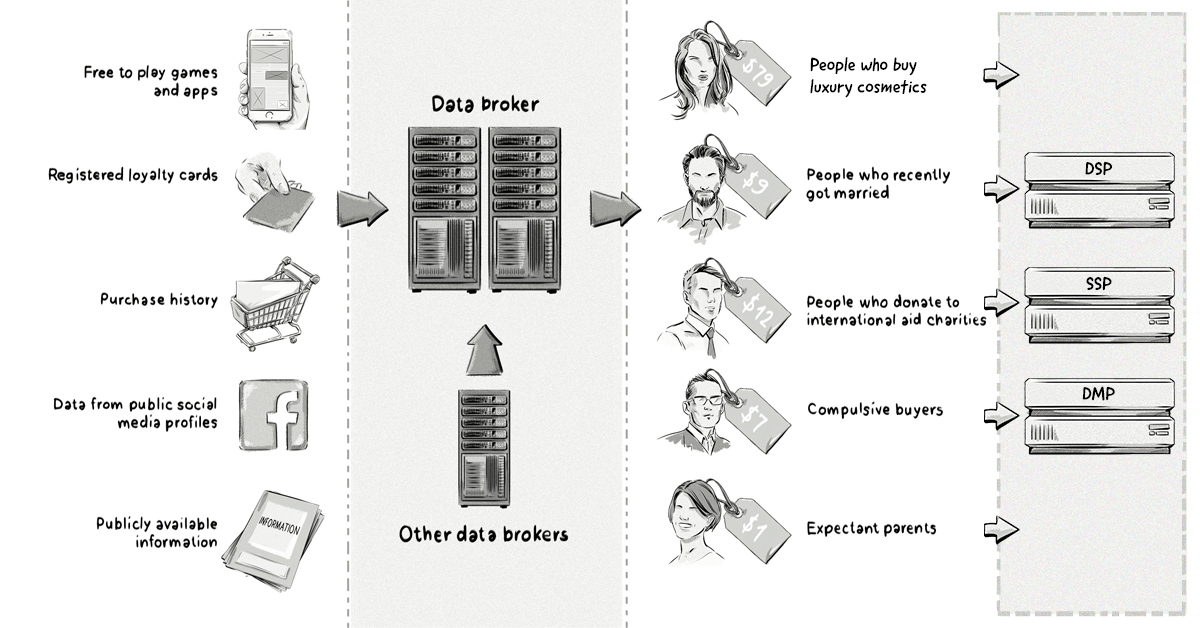
Some examples of data brokers in online advertising include:
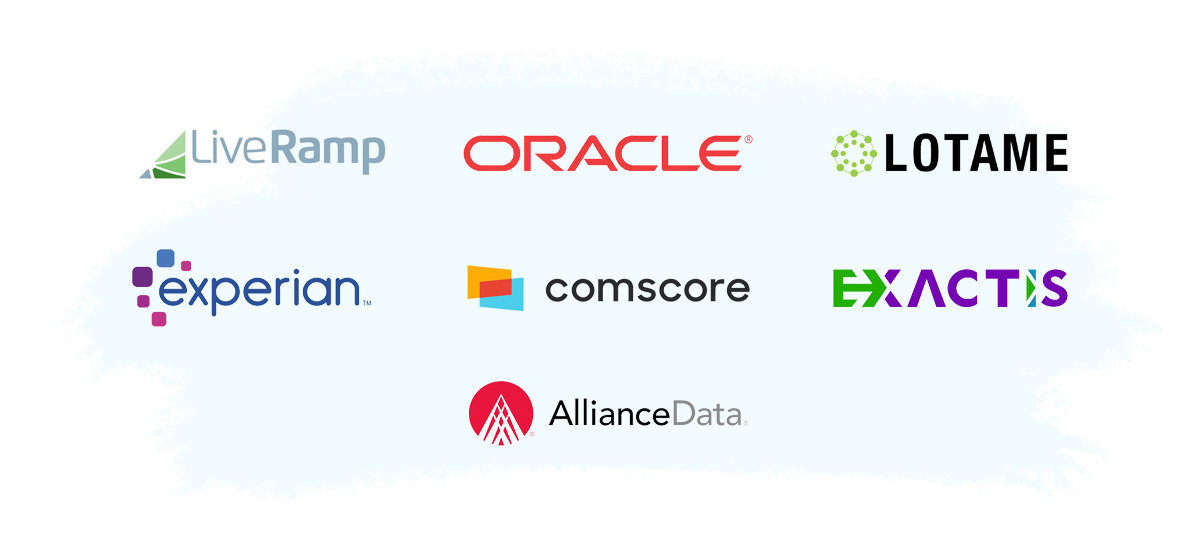
In the digital advertising and marketing industries, many DMPs act as data brokers and vice versa. The data collection process is similar to that of DMPs (listed above).
Data Pricing Models
Once DMPs and data brokers have collected the data, they usually sell it on to advertisers and AdTech vendors via two pricing models: a fixed CPM or a percentage of the media cost.
Fixed CPM Price
The most common way for DMPs and data brokers to sell data is on a cost per mille (cost per thousand) basis.
This means they are paid a fixed amount, such as $1.25 for each 1,000 unique cookies created by their site(s).
The main advantage of this model is that it provides a guaranteed price for both the data provider and advertiser/media buyer.
However, it doesn’t take into account important variables, such as context or the real value of the ad placement, meaning it places a fixed price on the audience which could actually be worth more than the CPM price suggests.
There are also issues surrounding the ROI of audience data sold on a fixed CPM price.
Often, the CPM price of audience data is actually more than the CPM price of the impression itself. This means that an advertiser could buy an impression on a CPM of $1.10 but the CPM of the audience data could be $1.25, which adds significant cost and makes generating a reasonable ROI much harder to achieve.
Percentage of the Media Cost
Many data brokers and advertisers/media buyers are moving towards a percentage of media cost pricing model due to the very issues the fixed CPM pricing model presents.
As the name suggests, data providers charge advertisers and media buyers a percentage of the media cost for their audience data.
So if the impression was sold at a CPM of $.50 and the data provider charged 20% of the media cost, then the advertiser or media buyer would pay $.10 for the audience data.
While it may appear that the data brokers is losing money via the % of media cost, they are actually making third-party data more valuable and useful for advertisers and media buyers as they benefit from improved targeting, optimized ROIs, and better campaign performance — all of which lead to high adoption rates of third-party data, which spells good news for data brokers.
The image below illustrates the cost differences associated with the fixed CPM pricing model and the % of media cost pricing model:
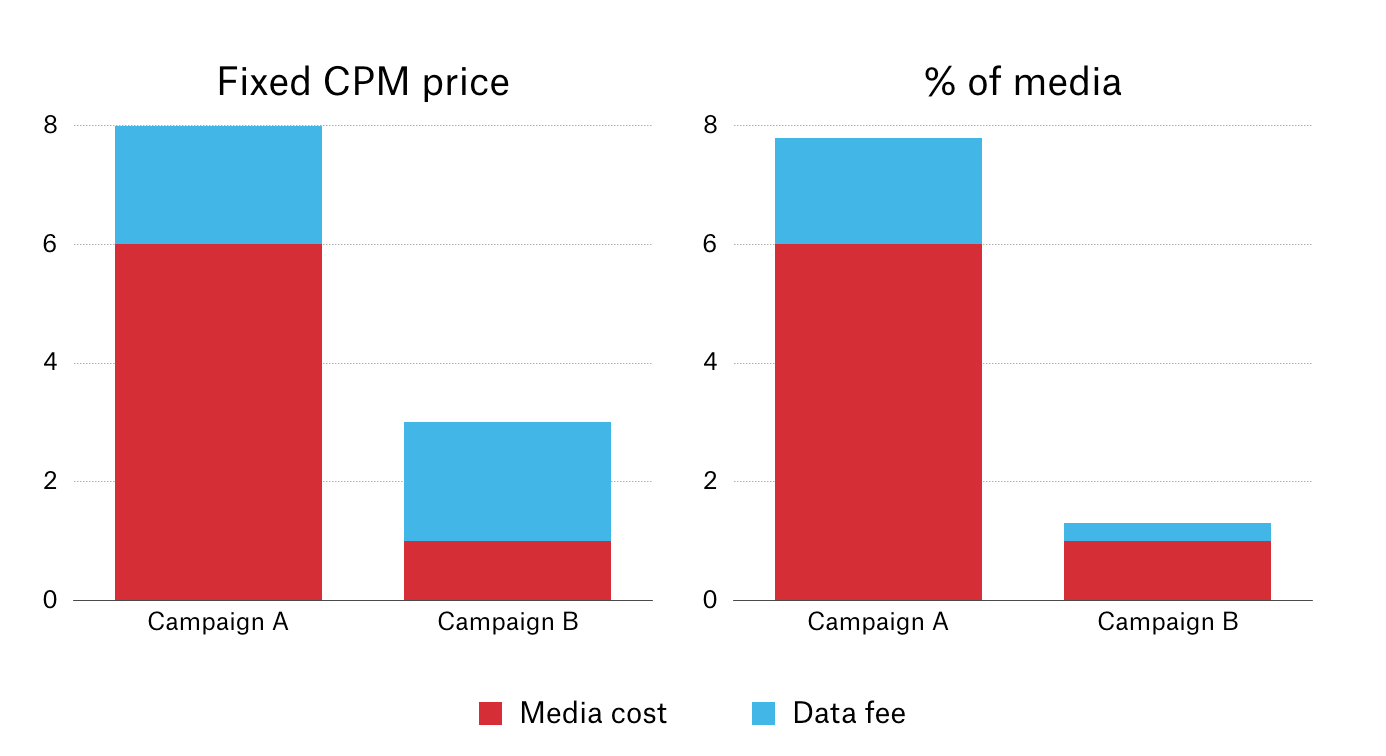
Some common problems with using third-party data in programmatic media buying:
Problem 1: The main issue with this method of selling through technology platforms is that it’s often hard to know whether a DSP used a certain audience from a DMP.
In the RTB auction model, data is usually provided in every bid request sent to the DSP. The bidder on DSP side sends bids on behalf of the advertiser, but there is no way to tell if the bidder used the data during the exchange.
Problem 2: The other problem with this model is that the price of the data is usually static.
The only difference is that some segments are considered premium or of higher value than others, and the CPM price is then higher. There is no way to dynamically set the price for the data based on the demand and/or quality, and therefore, all the parties in the ecosystem (e.g. publishers, data suppliers, data providers, advertisers, etc.) may be losing out financially.
The Future of DMPs
Because every digital advertising process is powered by data, DMPs have been a key component of a brand’s, agency’s, and publisher’s tech stack.
But the business model and future of many DMPs is in jeopardy because of the rise of privacy in AdTech.
Privacy laws like the GDPR, ad blockers, and privacy settings in web browsers are making it much harder to collect third-party data from websites.
Google Chrome’s announcement that they’ll be shutting off support for third-party cookies in 2024 means that almost 100% of web browser traffic won’t support third-party cookies.
To survive the next decade, most DMPs will need to look for ways to help publishers and advertisers identify their audiences without the use of third-party cookies, for example, by using identity resolution services.
Advertisers and publishers will also need to think about how they unlock the value of their first-party data.
In fact, this is already happening with the rise of customer data platforms (CDPs).
What Is a Customer Data Platform (CDP)?
A customer data platform is a piece of software that collects data from different sources and creates a single customer view of a customer, aka single customer view (SCV).
Although DMPs and CDPs are fairly similar in the way they collect, normalize, enrich, and activate data, there are a couple of main differences in the type of data they collect and how they use it.
As we mentioned above, DMPs typically collect third-party data and use it for advertising purposes. CDPs mainly collect first-party data and use it for multiple purposes, such as advertising, marketing, and customer support.
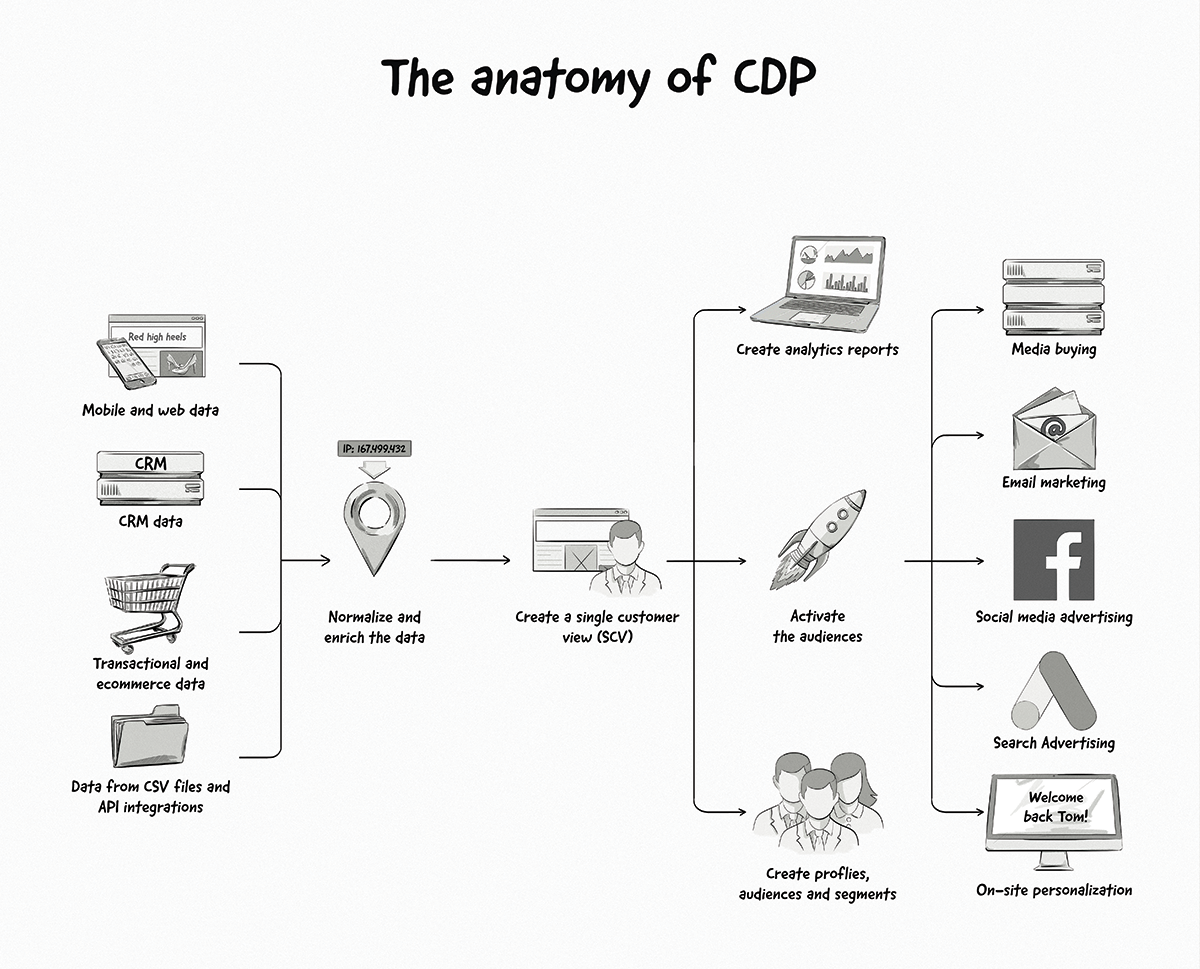
The rise of privacy laws and changes to web cookies has forced advertisers and publishers to put more focus on utilizing their first-party data.
Publishers, for example, can collect first-party data, create user profiles and audiences in a CDP, and offer those audiences to advertisers for ad targeting.
Now that we’ve had a detailed look at the role data plays in the online advertising ecosystem, it’s time to turn our attention to an area that heavily relies on data – attribution.
Test your knowledge with our quiz!
Download the PDF version of our AdTech Book
Read and download the PDF and register your interest for the hardcover version.
Download the PDF version of our AdTech Book
Fill in the form to download the PDF and join our AdTech Book email list to receive all future updated versions, including information about the release of the hardcover version.